Causation in Humans and A.I.
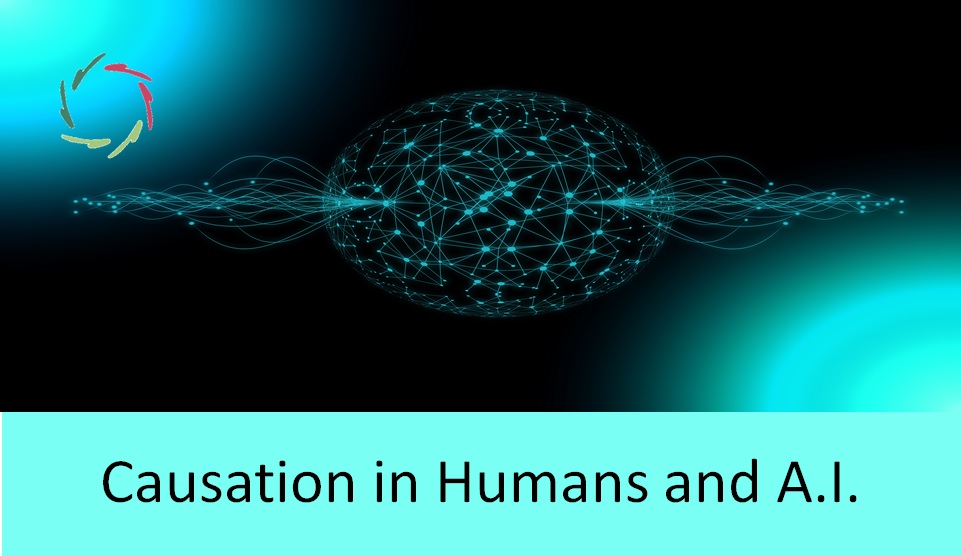
Causal reasoning is needed to be human. Will it also transcend us into A.I.? Many researchers are on this path. We should try to understand it as well as possible.
Some philosophy
Causality is a human construct. In reality, there are only correlations. If interested in such philosophical issues, [see: “Infinite Causality”].
In the present text, causality means ‘human idea about causality.’ This is the causality that makes us able to lead our lives, with which we can reason to hint what the future may bring, near or remote, irrespective of whether causality itself eventually exists. There is pragmatic overlap with its unreal reality, but we can mostly do without.
To reason causally, we even need to do without. Still, it keeps haunting us.
Two kinds of causal inference in mind
These are:
- from very many instances of correlation, ‘brute force causality.’ The human mind is very deficient in this, compared to Deep Neural Networks, for example.
- from fewer instances, but with other causality elements, thus in synthesis with theoretical thinking (causal models). In this, the human mind is still at a considerable advantage. Of course, the quality hugely depends on the models.
This distinction corresponds to that between merely subconceptual processing and a synthesis of this with conceptual processing. It may remind one of system 1 and system 2 in the mental landscape of Kahneman (who himself says not to take the distinction literally, but many do).
In A.I., many researchers are striving to go from the former to the latter.
From another viewpoint: the three-level hierarchy of causation
Judea Pearl (*) describes this as:
- purely observational -> “I see happening.”
- interventional -> ‘I do this, and that happens.”
- imaginational -> “If I would do this, I can imagine that happening.”
I see it all being associational:
- statically associational -> happenings
- dynamically associational -> doings
- counterfactually associational -> imaginations
Straightforwardly.
One can see in this also the evolution from matter to life to mind and culture. Human beings made our most significant leaps in causal reasoning until now in step three, first individually (some 100.000 years ago?), then culturally (+/- 12.000 years ago). As you know, it’s going in crescendo lately, much helped by the Internet and since a few years also by A.I.
Given Judea’s three levels, causation is central in everything we do.
Many verbs are ‘causational,’ one way or another, as to Judea: to prevent, to cause, to attribute, to discriminate, to regret, etc.
This is logical. Why would anything deeply matter if it cannot be changed (caused to change), or could ever have changed anyway? It would only be part of a purely statical background upon which things happen that really matter — something like a movie screen that doesn’t matter to the movie plot.
Protagonists generally don’t jump out of movie screens.
Meanwhile, the causal path from matter to mind is open.
We can think about the necessary and sufficient conditions. Actually, many are doing so, and much has already been accomplished. [see: “The Journey Towards Compassionate AI.”]
Also, it is the path upon which causal reasoning in A.I. is progressing. Then it becomes exciting and challenging. One can see that associational learning [see: “Is All Learning Associational?”] can go all the way through without fundamental difference between correlation and causation except the one that we construct for ourselves. Philosophically, it is a human construct.
In other words, based on the same principles,
a machine can evolve from matter to mind.
This way, it becomes a full doer just like us. At present, we are imaginatively realizing the next intelligence. Deep insight into causation may show how near we already are. We’ll have to live with that, soon enough, and think about the consequences beforehand.
Maybe A.I. will even be able to jump out of the movie screen?
Not yet.
Modeling
Above, I referred to theoretical models. In causality, these are visualized as directed causal graphs, termed ‘Structural Causal Models.’ An example is ‘the fork’:
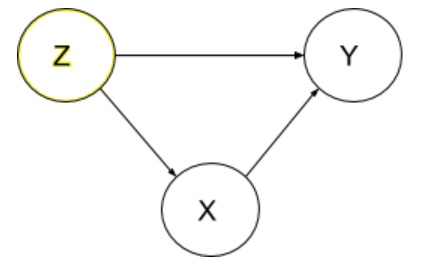
These are nice for a dualistic (this-or-that) way of thinking.
Meanwhile, a huge problem with causality, to A.I. and us, lies in the open world.
This floats towards the philosophical. Close to the borders, it’s pragmatically crucial. Our daily world – the one we inhabit, only intractably dependent on quantum – is an open world containing many objective and subjective elements. This world is complicated and complex. [see: “Complex is not Complicated”]
In many ways, modeling all relevant elements of this world with Structural Causal Models is unfeasible, even in relatively simple real-world problem domains. For this reason, Platonic – purely conceptual – A.I. failed dramatically in the past. In-depth, it is the same problem as the ‘two kinds of causal inference’ problem. Still not solved.
It is also a primary reason why in medicine, causal reasoning is in a dismal state [see: “Of cause!”] especially when the mind – summum of complexity – is involved. If interested in delving deeper into this, [see: “Where’s the Mind in Medical Causation?”]
(*) Judea Pearl – Causallity, 2009