Is All Learning Associational?
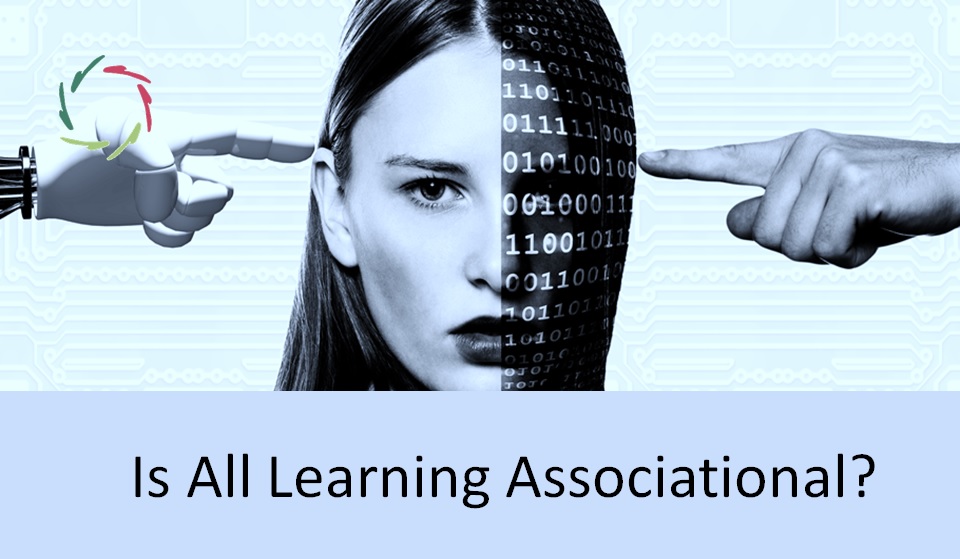
Most probably. This is a domain where animal/human learning and A.I. learning can learn much from each other.
Three forms of learning in A.I.
Generally, learning in A.I. is divided into two distinct kinds, with a third one dangling in the appendix, referring to another book (*).
- supervised learning: training with specific input and labeled output patterns
- unsupervised learning: The system has to discover the features of the input population on its own, no labeled output patterns.
- reinforcement learning: of actions towards maximizing the notion of cumulative reward
From three to one
A child sees a cow in a field. The mother points to it and says it’s a cow. This seems a prime example of supervised learning. However, at a higher plain, the child sees the cow and hears the word ‘cow’ from mommy. This is one pattern that the child encounters as a whole. As such, this pattern comes to the child as a whole. The child learns this pattern unsupervised.
In reinforcement learning, the patterns contain correlations (associations) with a payoff. You can also view this from a higher plain and again come to unsupervised learning.
More or less likewise, you can see in all three a kind of reinforcement learning, as well as a kind of supervised learning. Of course, there are differences between the three, but these are relative and gradual.
For completeness, there is also self-supervised learning, but this can easily be seen as unsupervised learning. It fits in our scheme of parsimoniousness ― one paradigm ruling them all.
And the winner is… associational learning (not surprisingly).
This is: associations between patterns. Some of these patterns are complex, others very simple. In some, there is the involvement of time; in others, not.
This coincides with the ubiquitous pattern-based processing in the brain. [see: “Human Brain: Giant Pattern Recognizer“]
So, the brain works in a basically uniform way: patterns and associations between patterns. When activated, the associations bring about transformations in the patterns. So much with so little,
Letting this winner win in the world
Now that we know that all learning is associational, we can try to make this as efficient as possible, as spontaneous as can get, with little ‘being done.’ This little is not, therefore, less important ― quite the contrary. It should be subtle and highly efficient in humans as well as A.I.
Does it sound a bit like the ancient Chinese ‘wu wei’ principle? Or you prefer ‘less is more’? Or ‘In der Beschränkung zeigt sich erst der Meister.’? It’s a general insight.
Most efficient = most spontaneous = most sustainable = most ethical = most healing in the broadest sense.
Associational learning in small steps
This is, in many cases, most efficient. Intelligent learning/teaching then consists in finding the optimal breakdown in steps of the right size and relevance, possibly combining bottom-up and top-down processing.
There is no cookbook to be made about the right kinds of steps. Some guidelines:
- In most cases, people are too much inclined towards supervised options. That may be most of all related to how consciousness evolutionarily evolved, in a natural search for modularity, then conceptualization. So, in most cases, try to relax supervision. In A.I., we see an exaggeration of supervised learning and a profound admonition from G. Hinton, for instance, to put the efforts much more in unsupervised learning. According to him, here lies the future of A.I. Note that our previous point of supervised/unsupervised is also acknowledged by Hinton.
- Towards humans, see yourself not as the one who uses instruments, but as the instrument itself ― for instance, in leadership or coaching. From deep to deep, associations are most proficient. In A.I., this translates into using much more parameters, fewer cycles of training. Handcrafting very many parameters may be pretty labor-intensive, which leads to a preference for unsupervised learning.
- Look not only at a superficial level for optimal levers. In coaching, this is ’empathy beyond.’ In A.I., this translates into a search for different levels between bottom-up and top-down, and a choice for the most promising one at short and long term.
- A yes-attitude is best in most cases. Try to find a level of depth at which the ‘yes’ is the right answer to the question you want to pose. In A.I., this is the very basis for the success of present-day Deep Neural Nets: their not getting stuck in local minima because of their multidimensional gradients in which there is always an optimal ‘yes gradient’ to be found.
Unfortunately, people are stuck on the idea that “it needs to be done.” That way, much learning is made much more difficult from the start. The learner gets demotivated ― as does the teacher. It goes from worse to terrible.
Autosuggestion
Much learning is on the nonconscious, subconceptual level, such as learning to quit smoking. This is associational as well. It can be transformational. Preferably, the patterns used are deep-level ones for the learning to be person-oriented, with huge advantages and effects also at the physical level. [see: “Autosuggestion Changes Your Brain“]
Suggestion is not merely informational, nor is it coercion, but a ‘touching of deeper patterns.’ You see the pattern level coming through, and the associational aspect within the ‘touching.’ It’s an invitation, an opening of doors for whom wants to go through them, and a showing that it’s OK, also why it’s OK.
In the end, it’s a special kind of associational learning.
To me, autosuggestion is but a language that one can use in many ways towards change of mind/brain patterns. AURELIS stands for a profoundly ethical and efficient way for many. By itself, the ‘auto’-part is ethical already: You do it yourself, from inside out.
This way, AURELIS is naturally growth-oriented.
(*) Probably the best is Reinforcement Learning – An Introduction, sec. ed. by Richard S. Sutton and Andrew G. Barto (2018). Please be motivated before you buy it. It’s a heavy ‘introduction.’