Is Lisa Safe?
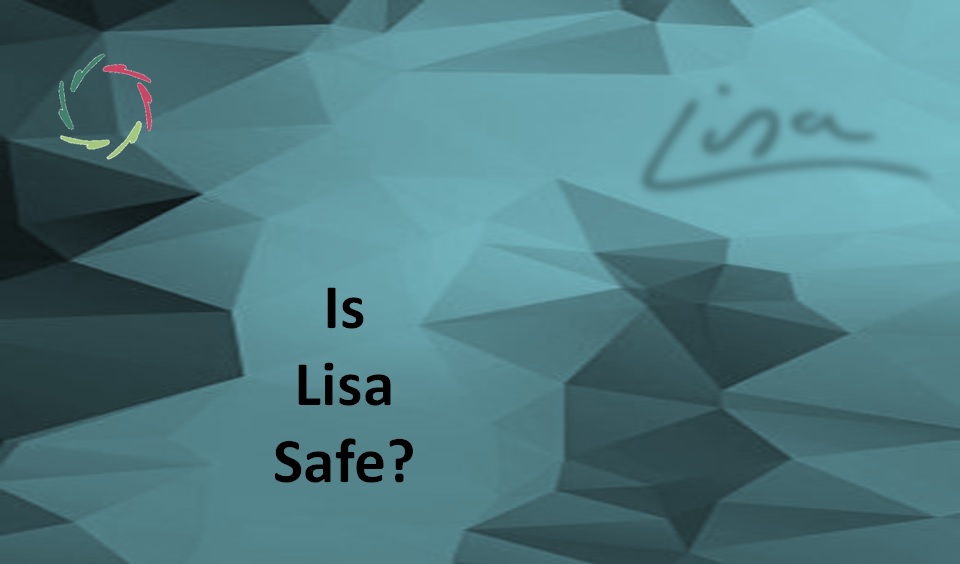
There are two directions of safety for complex A.I.-projects: general and particular. Lisa must forever conform to the highest standards in both.
Let’s assume Lisa becomes the immense success that she deserves. Lisa can then help many people in many ways and for a very long time — a millennium to start with.
About Lisa
If not already done, please meet video-coach-bot Lisa in development.
But this text is also about any truly complex A.I.-project now and in the future. When talking about Lisa today, I also talk about the latter.
This text is not an exhaustive exposé or ‘proof of safety.’ It gives some ideas that I deem noteworthy for Lisa and the domain in general. Also, this text has an explicitly deontological take. The juridico-technical take is additionally important.
Of course, we do our best to make Lisa safe.
Even that is not enough. The safety endeavor must include a continual effort to strive for optimal future safety.
That is not something to start thinking about when the stream gets rough. The stakes are too high.
Particular safety
An individual user might encounter a unique issue. In any such case, the system must be prepared to react appropriately.
No matter how many concrete rules we might put in place, this alone will never reach the desired safety level. People also don’t proceed this way for themselves. We use rules of thumb in many familiar situations ― mainly for speed and resource efficiency.
On top of that, we also use broader mental pattern recognition in safety issues and more. The procedure is to recognize clear patterns of danger, safety, and uncertainty. When safe, we relax; we go into stress mode when in danger.
The same applies to Lisa. The end goal is to play it safely and realistically.
This is why an overly stochastic system doesn’t pass muster ― as also a system that rashly proceeds with uncertainty patterns.
This means a hard job of human thinking, then of Lisa-aided human thinking. We don’t need to wait until everything is thought through, but enough to play safe before proceeding.
Particular safety is a lot of relevant work.
General safety
This is not about very specific issues. Therefore, it may need less of a monk’s work. It’s a different job, but a lot of it anyway. This is, for instance, about the system’s influence on sociocultural issues.
One should not see such issues as collateral damage or things to be solved by society in the ‘common domain’ that would, therefore, be hardly relevant to the specific project ― Lisa, in our case.
Contrary to this, it belongs to the project’s unmistakable responsibility.
The ‘solution’ is not, therefore, a clearcut magical key. It is rather a meaningful contribution, an openness to share insights and a never-ending willingness to ‘make this world a better place.’
This is not rule-based but intention-based. That doesn’t make it less realistically relevant ― quite the contrary. It does point to yet another essential change (of which there will be many in the A.I.-future): from rules and guilt to hands-on responsibility ― meaningfully based on the trust that people can be highly motivated by the latter. Fortunately, science robustly shows this to be the case IF people are not demotivated or ethically damaged.
With Lisa, we take this responsibility extremely seriously.
Explainability
In short, explainability is about the system’s ability to explain in commonly understandable terms why some decision has been made.
On top of general and particular safety, explainability is also something we must take very seriously at every step. The user may ask the system’s explanation for some specific decision, which must immediately be given or upgraded to developers. The human-thinking equivalent of the latter is when we run into some more challenging issue and need to take some time off to think about it, consult some other humans or books. The wise person knows when it’s time to do so. We need to put such wisdom into the system, which can be done pattern-wise.
The developers may also be asked for explanations for more general issues. Such explanations are to be viewed as part of the ‘product.’ They should be made publicly available.
This does not entail opening up every detail of the internal workings ― being commercially unrealistic. It does entail accountability of decisions, as one might ask of humans. No human knows the intricate details of personal decisions ― far from that. Nevertheless, the concept of accountability is viable and effective.
With explainability comes the possibility of co-creating safety by many users. Surely, this needs much common sense. It is one factor that becomes feasible in combination with others ― then also important.
In the very long term
Some people might mistrust super-A.I. by an unshakable principle. To be trustworthy, nevertheless, we put in place two more principles for Lisa safety also in the long term:
- There is a well-thought-out and documented ethical base. Very much work on this has been done.
- Lisa gets a detailed framework of human-understandable constraints forming the applicable mindscape. This is the top level of where all other inferencing (the ‘thinking’) gets done. This is also a strict part of the interface between the ‘Lisa mind’ and developers. It forms the consistent trustworthiness central to the ethical base ― thus, in accordance with everything else about Lisa.
Last but not least, we consistently choose for technologies that make this all feasible, already foreseeing specific proprietary upgrades. Progress is such that there are many relevant choices in this. This way, Lisa’s compassion is engrained to the core, and we can trust it to remain there.