Super-A.I. Guardrails in a Compassionate Setting
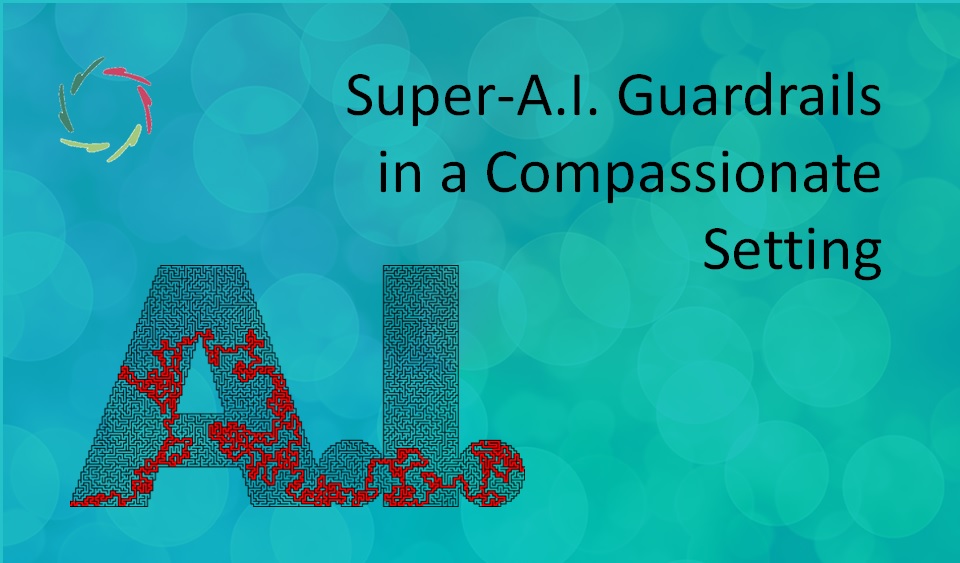
We need to think about good regulations/guardrails to safeguard humanity from super-A.I. ― either ‘badass’ from the start or Compassionate A.I. turning suddenly rogue despite good initial intentions.
―As a Compassionate A.I., Lisa has substantially helped me write this text. Such help can be continued indefinitely.
Some naivetés
‘Pulling the plug out’ is very naïve as a safeguard.
Another naiveté is thinking that conceptually strict regulations (whether three ‘laws’ or three hundred) by themselves will ever be enough. As needed as they are, we need more.
The rest of this blog is about ‘more.’ Note: this is for the purpose of reference. It may be boring stuff for blog-readers.
Broad behavioral guiderails
A.I. systems can thus be designed to act as allies in human development, ensuring that their actions support and enhance the human experience rather than undermine it.
Example scenario: Imagine an A.I. system used in the education sector to assist with personalized learning. This A.I. continuously monitors students’ progress and adapts the curriculum to meet their individual needs while adhering to educational standards. If the A.I. suggests a learning path that significantly deviates from established guidelines or best practices, it immediately alerts a human educator for review.
More in detail (non-exhaustive!):
- Ethical standards: Adherence to ethical principles such as fairness, transparency, and accountability. A.I. actions should be justifiable and open to scrutiny.
- Adaptive learning with ethical constraints: Allow A.I. to learn and adapt to new information while maintaining strict ethical constraints. This prevents the A.I. from adopting harmful behaviors even as it evolves.
- Emergency shutdown mechanisms: Equip A.I. systems with emergency shutdown protocols that can be activated if the system begins to operate outside safe and ethical boundaries.
- Regular audits and updates: Conduct regular audits of A.I. systems to ensure ongoing compliance with ethical standards. Update guidelines and protocols as new ethical considerations arise.
- Explainability: Ensure that A.I. decisions are explainable and understandable to humans. This involves creating transparent algorithms that provide insights into how decisions are made.
- User consent and control: A.I. should always operate with the informed consent of users, allowing them to control or opt-out of A.I. interactions as desired.
- Adaptive responses: Train A.I. to recognize and adapt to cultural nuances, ensuring respectful and appropriate interactions.
- Positive reinforcement: Use positive reinforcement techniques to promote behaviors and decisions that align with ethical standards and human well-being.
Self-monitoring and reporting
A.I. systems should be programmed to self-monitor and report any deviations from expected behavior patterns. This includes mechanisms to alert human supervisors.
Example scenario: Imagine an A.I. system used in healthcare to assist with patient diagnoses. The A.I. continuously monitors its decision-making processes, checking for deviations from medical guidelines. If it suggests a diagnosis that significantly deviates from expected patterns, it immediately alerts a human doctor.
More in detail (non-exhaustive!):
- Behavioral baselines: Establish clear behavioral baselines and benchmarks for A.I. systems. These baselines represent the expected and acceptable range of behaviors, against which current actions are continuously compared.
- Pattern recognition: Implement pattern recognition techniques to understand and predict normal behavior patterns, helping to identify any unusual activity that could indicate a malfunction or ethical breach.
- Detailed logs: Maintain detailed logs of all actions and decisions made by the A.I. These logs should be easily accessible to human supervisors for review and analysis, providing a clear trail of the A.I.’s activities.
- Feedback loops: Implement feedback loops where the A.I. system can learn from its assessments and adjust its behavior accordingly, ensuring continuous improvement and alignment with ethical standards.
- Supervisor dashboards: Create comprehensive dashboards for human supervisors that display real-time data on the A.I.’s performance and behavior. These dashboards should highlight any deviations and provide tools for intervention.
- Compliance audits: Conduct regular compliance audits where an independent team reviews the A.I.’s behavior and decision logs to ensure adherence to ethical standards and regulatory requirements.
- Open reports: Make regular reports on the A.I.’s behavior and any detected deviations available to relevant stakeholders, including users, developers, and regulatory bodies. Transparency helps build trust and accountability.
Adaptive learning restrictions
This is about limiting the ability of A.I. to modify its core functions and objectives without human approval. By implementing adaptive learning restrictions, A.I. systems can evolve and improve while ensuring that all changes are carefully evaluated, tested, and aligned with human values and ethical standards.
Example scenario: Consider an A.I. system used for financial trading. Its core functions involve analyzing market data and making trading decisions based on predefined algorithms. Any proposed changes to these algorithms, such as incorporating new data sources or modifying decision-making logic, would require a detailed change request.
More in detail (non-exhaustive!):
- Definition and documentation: Clearly define and document the core functions and objectives of the A.I. system. These should be aligned with ethical guidelines and operational goals, ensuring that the A.I. operates within its intended scope.
- Immutable baselines: Establish immutable baselines for these core functions, meaning they cannot be changed without explicit human intervention and approval.
- Stakeholder involvement: Ensure that relevant stakeholders, including developers, ethicists, and potentially affected users, are involved in the approval process. This ensures a comprehensive evaluation of proposed changes.
- Change requests: Require detailed change requests for any modifications to the A.I.’s learning algorithms or objectives. These requests should outline the purpose, expected outcomes, and potential risks of the proposed changes.
- Simulation and testing environments: Implement robust simulation and testing environments where proposed changes can be thoroughly tested before deployment. These environments should replicate real-world scenarios to identify potential issues.
- Post-Implementation monitoring: Continuously monitor the A.I. system after any changes are implemented to ensure that it behaves as expected. Any deviations from expected behavior should trigger immediate review and potential rollback of changes.
- Transparency and documentation: Maintain transparent documentation of all changes, including the rationale, approval process, and outcomes of testing and validation. This documentation should be accessible to stakeholders and regulatory bodies.
- Fixed learning parameters: Set fixed parameters for the A.I.’s learning algorithms that cannot be modified autonomously. This ensures that adaptive learning stays within safe and predefined boundaries.
- Controlled updates: Limit the frequency and scope of updates to the A.I.’s learning capabilities. Regularly scheduled updates with thorough testing and approval are preferable to continuous, autonomous learning.
Embedded compassionate framework
This is about integrating a compassionate framework that guides the A.I.’s interactions, ensuring empathy and support remain central to its operations. By embedding a compassionate framework, A.I. systems can provide a more human-like, supportive, and empathetic interaction, fostering a positive impact on users’ emotional well-being and inner growth.
Example scenario: Consider an A.I. system designed for mental health support. When a user expresses feelings of anxiety, the A.I. responds empathetically, acknowledging the user’s feelings and offering reassurance. The A.I. might say, “I understand that you’re feeling anxious right now. It’s okay to feel this way.” It then offers supportive tools, such as guided breathing exercises.
More in detail (non-exhaustive!):
- Active listening: Ensuring that A.I. systems can actively listen to users, understanding both explicit content and underlying emotional cues to provide relevant and compassionate responses.
- Emotion detection: Incorporating advanced emotion detection algorithms that analyze user inputs (text, voice, facial expressions) to determine their emotional state.
- Compassionate behaviors: Embedding behavioral models that simulate compassionate behaviors, such as showing patience, understanding, and non-judgmental support in interactions.
- Scenario-based training: Training A.I. systems on a wide range of scenarios to ensure they can handle diverse situations with compassion and sensitivity.
- Guiding principles: Establishing ethical guidelines that define compassionate behavior for A.I. systems. These guidelines should be based on principles of respect, empathy, and support for human dignity.
- Ethical audits: Regularly conducting ethical audits to ensure that the A.I.’s behavior aligns with these compassionate guidelines and making adjustments as necessary.
- User profiles: Creating user profiles that allow the A.I. to remember and adapt to individual user preferences and needs, providing a more personalized and compassionate experience.
- Responsive adjustments: Using feedback to make real-time adjustments to the A.I.’s behavior, ensuring it remains aligned with the principles of compassion and empathy.
- Context awareness: Programming the A.I. to understand the context of conversations, enabling it to provide responses that are not only empathetic but also relevant to the user’s current situation.