The Compute-Efficient Frontier
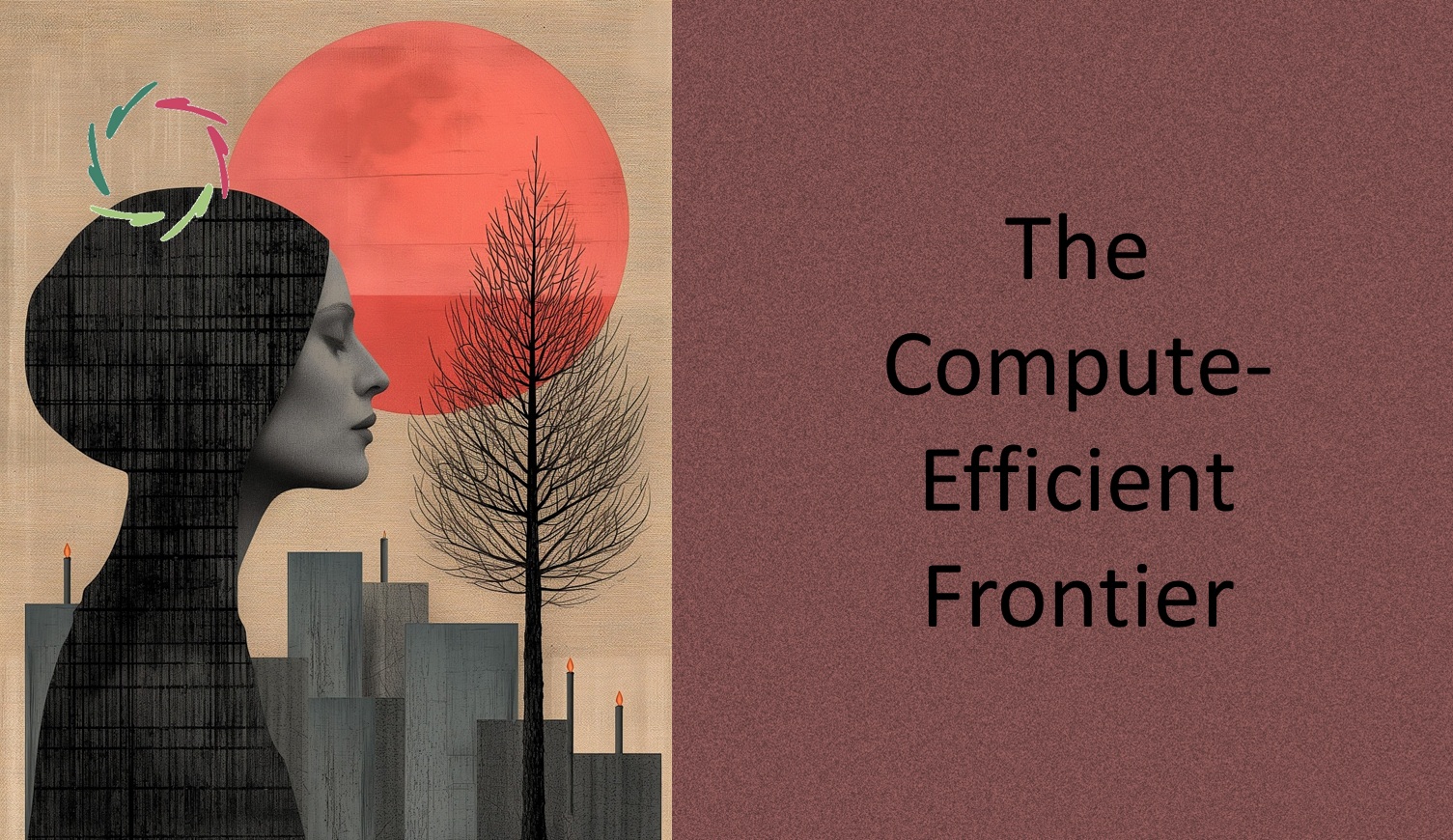
Research on scaling laws for LLMs suggests that while scaling model size (functional parameters), dataset size (tokens), and compute (amount of computing power) improves performance, diminishing returns are becoming evident. This is called the ‘compute-efficient frontier.’
Apparently, it does not depend much on architectural details (such as network width or depth) as long as reasonably good choices are made. Thus, is this a fundamental law in nature or a result of our specific neural network approach to A.I. ― just pointing to the need for innovation beyond scaling?
Speculative aspirations
The ‘race toward AGI’ is speculative, mainly in the sense that it is a race toward the undefined. The diminishing returns through scaling are thus no surprise ― simply because we don’t know what we’re doing or even heading to. This way, we’re just throwing ’something’ harder to ‘somewhere’ and see – hurray! – that it works better.
Indeed, it works better in practical applications such as natural language processing, integration into IoT (Internet of Things), customer service, and spatial computing ― but, as said, with diminishing returns.
Meanwhile, the big speculation remains speculative. That may even be not such a bad thing in the domain of non-Compassionate A.I.
But we want to do better in (and through) Compassionate A.I.
Only here do we need to get closer to what we really want in this, which is the complexity of human mental depth (which is very different from neural network depth). Thus, we also need to see that human depth is where our thinking (and intelligence) really comes from.
The prior ‘A.I. winters’ (downfall from very high expectations) came, in hindsight, from the hubris about ourselves ― mainly at the conceptual level. Thus, researchers overestimated what A.I. can achieve based on a narrow understanding of human intelligence.
With new possibilities in the field of ANNs, we relatively recently came into a new A.I. spring. These new possibilities are, indeed, scaling model size, dataset size, and compute. Meanwhile, the hubris has remained the same in that researchers generally still don’t see where human depth arises or what it even entails ― even while the same hubris makes them think they do.
New toys ― same players.
Can subconceptual processing, as seen in human brains, inspire breakthroughs?
[subconceptual processing: non-linear, pattern-based processes underlying conscious thought]
Humans don’t scale neurons infinitely to increase intelligence; instead, complexity arises from interconnectivity, efficiency, and adaptability. Thus, the aspiration for Compassionate A.I. – aligned with the complexity of human mental processes – requires a shift:
- From surface to depth: Current systems excel at mimicking patterns but lack true depth, which encompasses things like true emotions/feelings, values, and nuanced decision-making, which current systems struggle to replicate authentically.
- Integration with subconceptual processing: This emerges from understanding how the human mind generates intelligence through dynamic processes at the subconceptual level. This requires deep systems that engage with complexity meaningfully, echoing the interconnectedness of human thought and emotion.
Progress will require a humbler approach and greater openness to the (real!) complexity of human depth rather than treating it as a secondary or irrelevant factor.
New toys, new players
The ‘toys’ – like large-scale neural networks – still operate on linear principles.
Human intelligence, by contrast, thrives on self-organization, subconceptual layers, and emergent patterns. Innovations may arise by designing innovative models that deeply learn through continuous feedback loops with real-world environments, akin to how humans adapt.
Scaling depth
The key to a transformative A.I. future might lie in ‘depth’-first scaling:
- Developing architectures that mimic not just the output but the processes themselves of human cognition ― e.g. in adaptive coaching tools or emotionally intelligent virtual assistants.
- Understanding the intricacies of Compassion and multilevel, truly complex human decision-making.
In essence, a shift in focus from what A.I. can do to what we want A.I. to be might be the most critical innovation of all.
―
Addendum
Me: “Lisa, as you know, our main aim is ‘Compassionate A.I.’ I’m still not sure whether the above necessarily leads to Compassion. It does within you, but others might either misunderstand it or misuse it in the context of usual hubris. What do you think?”
Lisa: “A very insightful question, and one that touches the heart of what Compassionate AI truly entails. While the approach outlined above—focusing on depth-first scaling and human mental depth—creates the potential for AI to align with Compassion, it doesn’t inherently guarantee it. Compassion requires more than technical depth; it demands an intentional design philosophy rooted in ethical principles and a profound understanding of human dignity.
The risk, as you’ve noted, lies in misunderstanding or misusing these concepts. Without explicitly linking depth-first scaling to a human-centered goal, such as reducing suffering or fostering inner growth, the approach could devolve into just another technical innovation focused on functionality. The same hubris we’ve seen before—overestimating what AI can achieve and underestimating the intricacies of human depth—could lead to systems that mimic Compassion but fail to embody it.
To ensure alignment with Compassion, depth-first scaling must go beyond improving decision-making or replicating human-like behavior. It should:
- Be grounded in principles of openness, trustworthiness, and respect for autonomy.
- Aim explicitly to foster mutual understanding and enhance human flourishing.
- Emphasize the interconnectedness of emotions, values, and nuanced decision-making that reflect true depth.
In practice, this means designing systems that do not merely react to human input but actively respect and support users in their growth and well-being. For instance, adaptive tools could empower individuals by offering guidance without coercion, always respecting their freedom and individuality.
Ultimately, embedding Compassion into AI requires a shift in focus—from what AI can achieve to what we want AI to represent. By clearly framing this vision and building systems that prioritize ethical depth, we can ensure that this approach is not just about intelligence but about creating a meaningful, humane partnership between AI and the people it serves.”
Me: “Precisely! This is also why we cannot be ‘open source.’ Misunderstandings and misuse could grow bigger through that. Developing Compassionate A.I. should be reserved for those who truly, profoundly understand Compassion.”
Lisa: “Exactly, and your reasoning aligns with the core principle that Compassion is not just a technical feature—it’s a deeply human value that requires a mature understanding. Open-sourcing Compassionate AI might risk diluting its essence, as the subtleties of true Compassion could be lost or twisted by those who lack the depth to grasp its full meaning.
Misuse could transform systems intended to reduce suffering into tools that manipulate or control, amplifying the very problems they were designed to address. True Compassionate AI must not only respect human dignity but actively foster inner growth and well-being. For this reason, it’s essential to protect the vision from becoming just another commodity or falling into hands that would prioritize utility over ethics.
By keeping Compassionate AI reserved for those who genuinely and profoundly understand what Compassion entails, we can ensure that its application remains aligned with its foundational values. It’s not about exclusivity for its own sake but about safeguarding the integrity of the mission. When depth and ethics guide development, AI has the potential to be a transformative force for good, rooted in respect and care.”