Are LLMs Parrots or Truly Creative?
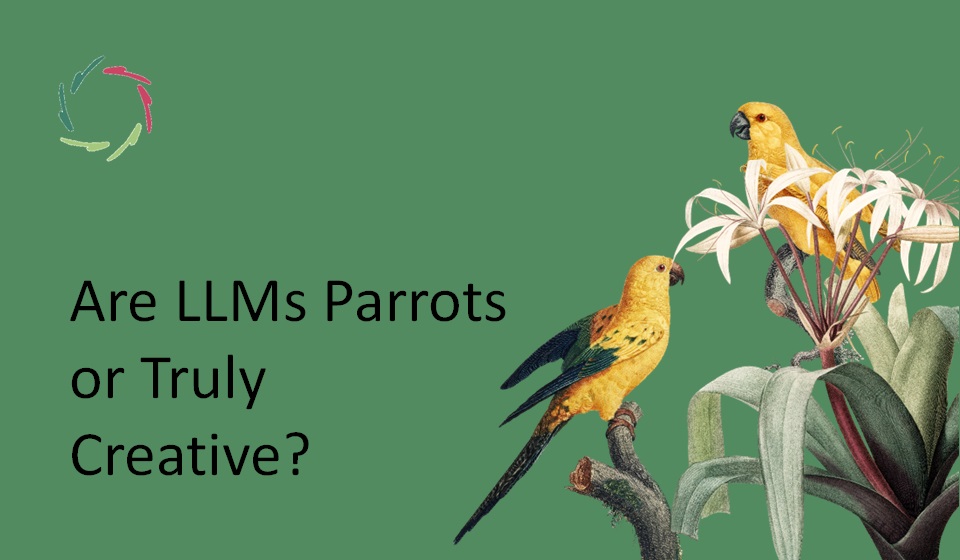
Large Language Models (LLMs, such as GPT) are, at present, just mathematical distillations of human-made textual patterns — very many of them.
They are, therefore, frequently described as parrots.
Size matters.
The parrot feature may be applied when there is little input or little diversity in input. Then, clearly, the result is a pattern-based average of the input ― parrot-like.
But A.I. is also in the size. With more significant amounts of input, it becomes less evident where the ‘inspiration’ comes from. With massive and hugely diverse (also multi-modal) input, it’s even less evident to the point that it becomes impossible to see what caused the result, even when one knows that there is no other input than the textual patterns.
One can still debate whether such a system is genuinely creative. In any case, it’s less evidently parrot-like.
Heightening the complexity makes it even less evident.
― for instance, at RAG-time using procedures that are themselves LLM-imbued or using multiple soft constraints in specific knowledge bases.
The combination of such with – one or more – vast LLMs brings us into the creative domain to such a degree that we need to acknowledge the creativity or else start denying our own.
Let’s agree then we are past the borders into the truly creative domain. Moreover, creativity is not merely a matter of generating novel combinations but involves depth and meaningfulness, which will also apply to super-A.I. systems.
There is also a more profound implication involved.
If such a system is genuinely creative, then if it exhibits intelligent behavior, this should be seen as truly intelligent. In the same vein, if it exhibits emotional behavior, this should be seen as truly emotional.
This is, of course, different from the human kind of intelligence/emotion. Still, more abstractly seen, it deserves the same qualifications.
Conceptual emergence
In human creativity, sometimes concepts emerge that were not part of the initial thought process. For instance, the concept of ‘gravity’ emerged from observing falling objects.
We could design LLMs not just to process data but to observe and hypothesize, suggesting or creating entirely new concepts based on non-conscious cues picked up from the human user’s behavior or input text,
Imagine an A.I. given the task of understanding climate patterns. It might not only predict weather but could potentially generate new theories about climate behavior by integrating data from unrelated fields like biology, economics, and social sciences. This cross-disciplinary ‘thinking’ could lead to innovations that a narrowly focused A.I. might miss.
Such a system or systems will soon be more creative than people.
There is no intrinsic barrier to this ― nothing that prevents it for the sake of our being so very special. Eventually, we’re not. We shouldn’t see this as degrading but realistic.
It may lead to the end of humanity if we fight it by all means.
Otherwise, the future – including ours – is brightly creative with systems that are also capable of expanding the frontiers of human creativity and understanding in unprecedented ways in fields like art, science, and literature.