More about Rewards (also in A.I.)
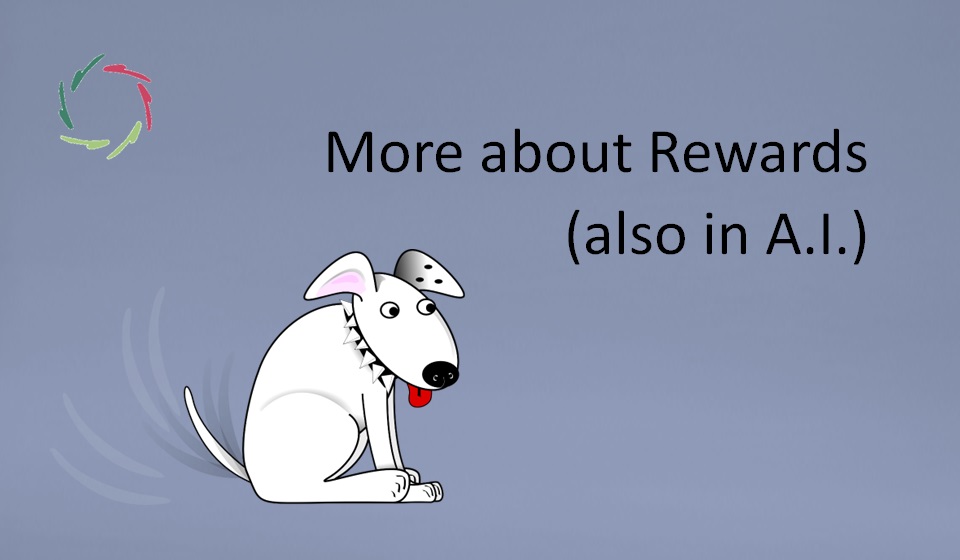
A reward is a nudge – with more or less lasting result – into some preferred direction. Anything can be experienced as a reward. Thinking about it as a pattern within a broader pattern is clarifying.
Pattern recognition and completion (PRC)
Seeing rewards in the context of PRC, a reward is always just a part of the observation of reality ― thus, not of reality itself, as explained in Agents and Expected Rewards.
Thus, the reward doesn’t need to be explicitly posited. Not doing so gives more freedom to the ‘rewarded’ agent to react in more freedom, in unexpected ways that may eventually also be more efficient.
The human case
Thinking about a reward as a pattern within a broader pattern corresponds with how the brain works as a predictor. The ‘prize’ then becomes the completion of the broader pattern.
Brain happy ― you happy.
Note that this can make one vulnerable to ‘rewards’ that are not necessarily in one’s best interest ― for instance, the woman repeatedly choosing the bad guy. Anyway, inside the brain, dopamine flows in expectation of the reward, which can always be seen as some form of PRC.
A.I. Reinforcement Learning
Reinforcement Learning (R.L.) is already an important part of A.I.. It is bound to become an even (much) more important part. Contrary to other A.I. technologies, it deals with simultaneously sequential, stochastic/evaluative, and partially observed reality (to be read twice, if you like). Within this, R.L. is about seeking a good policy (or strategy) to tackle pertinent problems.
Whereas other A.I. is about solving problems, R.L. is about developing strategies to solve problems (and standard software is about making the tools for solving them). Of course, R.L. can incorporate other A.I. technologies (for instance, DNN in Deep R.L.) and the needed software to make it all happen.
Sorry for this explanation at the sideline. It is needed to see where the rewards fit in.
Strategies are developed in R.L. by making an agent (either in software or as a three-dimensional robot) that can roam about and gather experiences. This is: it observes its environment, including what it is being given as rewards (positive or negative). Rewards are the food that R.L. needs to form its policies, from state to state and action to action.
Simply said, give it some rewards, and it will like you ― asking for more.
An indirect reward is an (auto)suggestion.
Instead of a direct command or a direct saying what is a good or bad move, an indirect ‘reward’ lends more freedom to the rewarded agent, also if it’s software. This has already been shown to lead to better results in most cases. The A.I. finds its own ways toward the preferred strategy. Not even formalizing what is or isn’t a reward gives it one more degree of freedom to look for the ideal solution.
Of course, this means that one needs to think through very well what one wants the system to achieve. This also pushes us toward thinking through very well what the final goals may be ― what our final goals may be.
Unfortunately, there is still much to be done concerning the latter.
We need to do that now.