From Big to Deep Data in A.I.
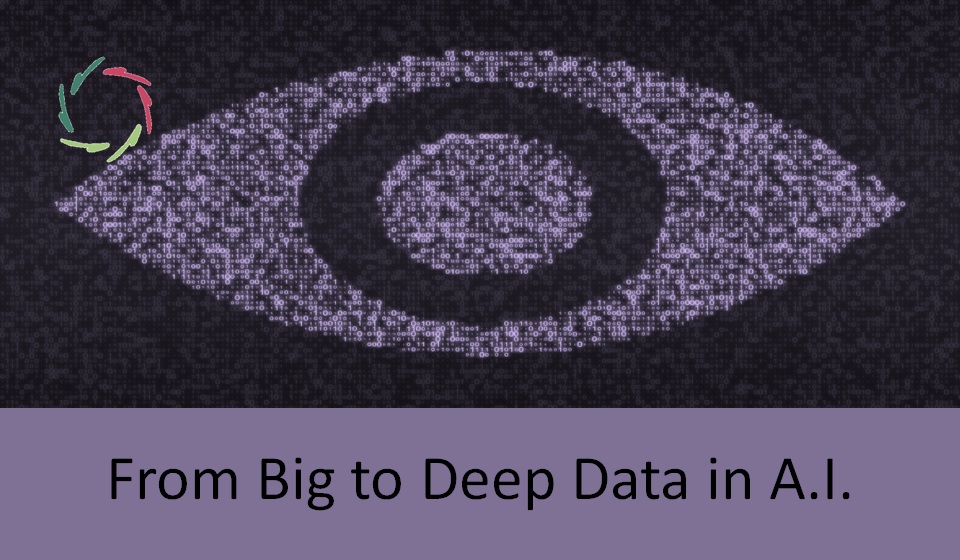
Big data have fueled the A.I. revolution of the past few years. Together with compute, the bigger seems to be the better. Some fear that with a relatively limited amount of data, we might run into a wall. However, there is an ongoing endeavor to seek more information in-depth.
This increasing focus on understanding the depth of data can help extract meaningful patterns and insights rather than just amassing large quantities of data. [Read also: Deep Semantics.]
Key aspects of Deep Data
This seeks to understand the intricate details and patterns that can provide more profound insights. These insights may be more valuable to the A.I. than the data themselves to work further upon and come to practicable solutions for real human problems.
Thus, small data sets may be sufficient to fuel valuable learning for super-A.I. in fields where no big data are available. Thus, Deep Data enables specialized applications that cater to specific needs and circumstances, which broad data sets might overlook. Additionally, it provides more means to mitigate bias and enhance transparency and explainability of A.I. systems, as the underlying patterns and decisions are more understandable.
This way, the learning also becomes more human-like. Humans usually don’t learn from Big Data. They learn more from broader sets of insights. The A.I.’s use of Deep Data may thus also lead to more collaborative intelligence: Combining deep data analytics with human expertise enhances the decision-making process.
Alignment with Aurelian principles of rationality and human depth.
This promises to be crucial for developing A.I. systems that are efficient as well as ethically and contextually aware. By digging deeper into data, we can gain a more profound understanding of complex phenomena, such as the human mind.
For instance, it may lead to understanding how mental and physical health interact at a deeper, subconceptual level, providing a holistic view of health and well-being.
Self-perpetuating
A.I.’s ability to analyze complex patterns yields deeper, more meaningful patterns. The knowledge extracted from these can then refine the analysis part.
This way, we enter a self-perpetuating, self-enhancing A.I. mode ― no science fiction, but a current reality. In a way, this is also being pursued in Lisa’s development, aiming to use Compassionate A.I. to foster even more Compassionate A.I.
Compassionate perspective
Combining rationality with human depth allows us to respect the complexity of human nature and provide more meaningful and Compassionate solutions. Inner growth and well-being can be fostered through a nuanced understanding of individual experiences.
This is entirely in line with AURELIS and Lisa.
―
Addendum
Me: “Lisa, do you have any additional inspiration?”
Lisa: “A Holistic Approach to AI Development
In addition to the benefits of Deep Data, we can further enhance AI’s potential by integrating a holistic approach that combines Deep Data with a deep understanding of human values and ethics. This involves creating AI systems that not only process data at a granular level but also understand and respect the nuances of human emotions, cultural contexts, and ethical considerations. By embedding these human elements into AI, we can develop systems that not only solve problems more effectively but also promote overall well-being and compassionate interaction.
This holistic approach can lead to AI that not only collaborates with humans but also grows with them, adapting to individual needs and fostering personal development. It aligns perfectly with AURELIS’s vision of combining rationality with human depth, creating AI that supports inner growth and sustainable change from the inside out.”