Analogy ― Last Frontier in A.I.?
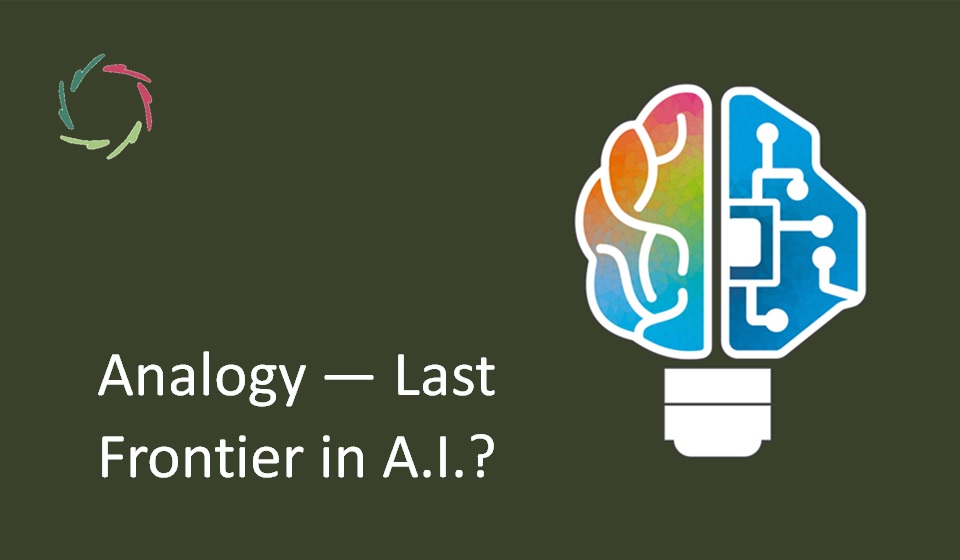
Big data, hugely efficient algorithms and immense computing power lead to present-day successes in A.I. Significant hurdles remain in learning from few occurrences and bringing to bear in one domain what has been learned in another ― thus accomplishing more general intelligence. Central to both is the use of analogy.
Humans are analogists
From childhood onwards, humans learn by analogy.
With a few similar experiences, a child can get the similarity and learn something new, such as “throw your toy on the ground, and mom will fetch it and give it back to you.” The child might even throw the toy on the ground again to get this familiar feeling of mom’s doing: “Hey, this works! And ow, it doesn’t work with dad. May some crying do the trick? Nope.”
Over a lifetime, humans frequently make sense of novel situations by making analogies with already known ones. This may lead to knowledge transfer (features in relationships) from the latter to the former, and an almost borderless intelligence.
‘Instant’ learning by analogy
With thinking by analogy, one occurrence in a different domain may already suffice to form and reason with a new concept. In A.I., this stands in stark contrast with supervised learning, where, in most cases, many thousands or more occurrences are needed for learning any new piece of knowledge: “This is a cat, not a dog.” Even so, the acquired knowledge remains brittle and with little generalizability. Each piece stays in its silo, like a dumb part of a genius.
Contrary to this, repetitive recognition of the same on different items or situations leads to abstraction and categorization. A new concept is born ― for instance, all gray things with twisted edges. As you can see, the new concept may or may not be relevant enough for broader use. Welcome to humanity’s history.
Abstract thinking
Humans tend to understand abstract concepts through analogy with concrete issues ― called ‘metaphors,’ not only in science but equally in daily life that is full of creative use of metaphors.
For instance, you can walk through this text or jump to the end.
Abstract thinking in A.I.
A.I. doesn’t know these physicalities first-hand but can learn them from us. With analogic thinking, it can form its own metaphors in abundance. Doing so, it can also use metaphors to jumpstart comprehension of its own thinking. Not much is needed to accomplish this.
In that case, ‘super-A.I.’ (real intelligence) can understand its human-embedded goals, think about them, and make them its own. It then ‘knows what it wants.’ In short, this way, it can proceed toward artificial consciousness.
There is little concern about this because there is little comprehension.
Analogy in complexity.
Analogy in a toy environment is easy. A complex environment makes it challenging because choices need to be made as to which aspects are essential to the analogy and which must be discarded to make the analogy ‘work.’
Within the complexity resides a pattern that needs to be recognized and completed ― whether implicitly or explicitly.
Humans make analogies with little effort because of the way our brain works in subconceptual processing. In A.I., we can either simulate this or start from scratch. Probably the shortest way is by simulating the brain while – indeed – abstracting what happens here to put it into practice there while making use of the specific features.
We are close.
The worst we can do is stop and let rogue developers be first. There is amazingly little concern about this because there is still amazingly little comprehension.
Honestly, is this another example of active basic denial?