Issues of Internal Representation in A.I.
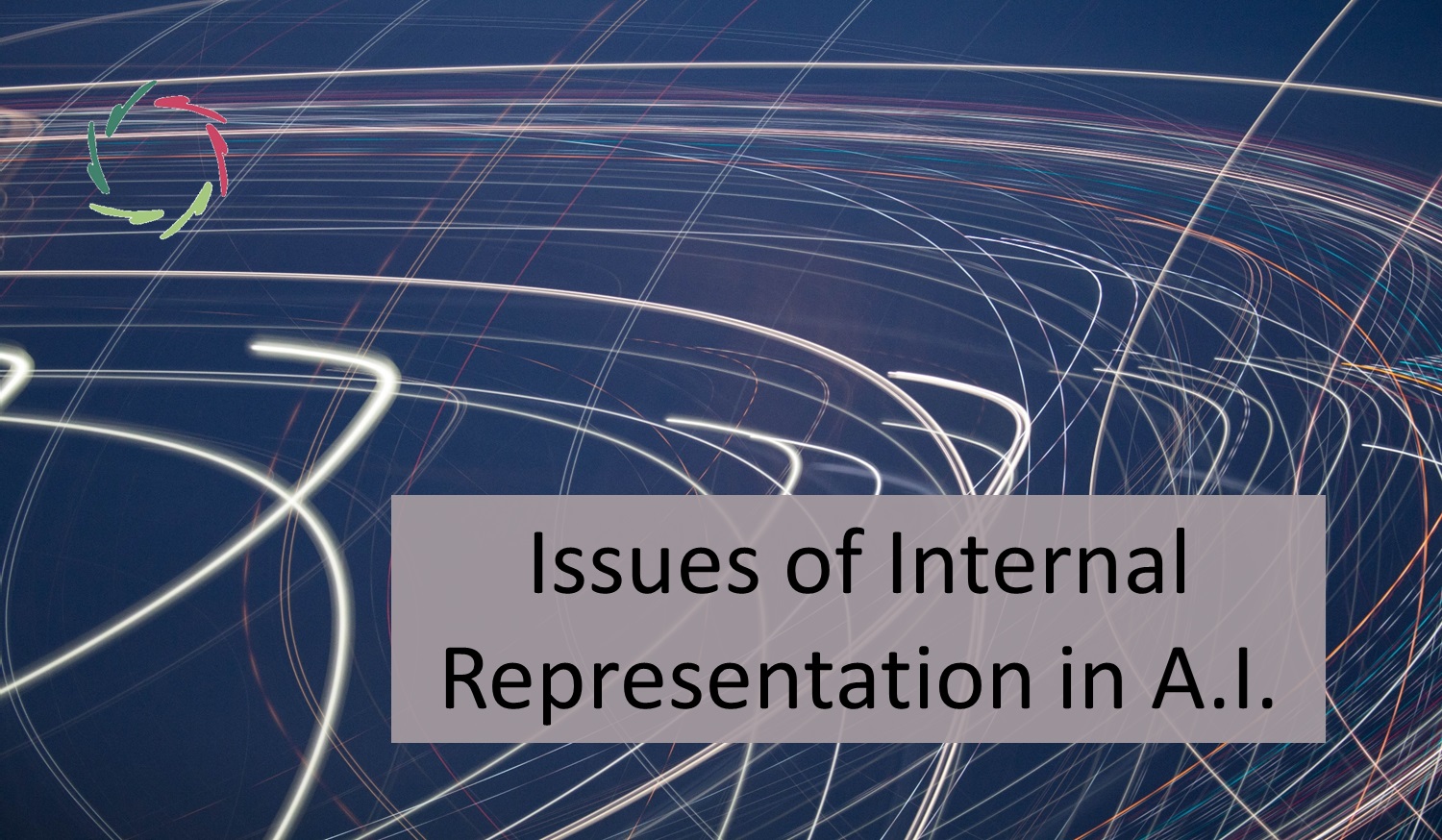
This is likely the most challenging aspect of developing the conceptual layer for any super-A.I. system, especially considering the complexity of reality and the fluid nature of concepts.
Representing conceptual information requires an approach that honors cognitive flexibility, contextual awareness, and adaptability. The model should allow for representational fluidity while maintaining enough structure to be useful in practical problem-solving and communication.
[NOTE: This blog is quite dry and might not interest the average reader.]
The following is a list of representational challenges:
Rigidity of concepts
- Challenge: Once a concept is formed, it can become too rigid, limiting the ability to adapt when new information arises. Concepts that don’t evolve can lead to misrepresentation of reality over time.
- Problem: How do we maintain fluidity and adaptability in representations to reflect changes in context or understanding?
Context sensitivity
- Challenge: Concepts are often highly context-dependent, meaning that their meaning can shift based on the situation or environment. A single representation might fail to capture the nuances of different contexts.
- Problem: How do we develop context-aware representations that can dynamically adjust based on the scenario without overcomplicating the system?
Subconceptual-conceptual balance
- Challenge: Representations need to bridge the gap between subtle, subconceptual insights and clear, conceptual knowledge. If we overemphasize one layer, we risk losing the richness of the other.
- Problem: How do we create a continuum of representation that allows for fluid transitions between subconceptual intuitions and structured conceptual clarity?
Handling ambiguity
- Challenge: Concepts can often be ambiguous and multi-layered, especially when they originate from complex, real-world phenomena. Simple representations may not capture this.
- Problem: How do we represent concepts in a way that embraces ambiguity without sacrificing the clarity needed for effective communication and decision-making?
Overconfidence in representations
- Challenge: There is a risk of assuming that a representation is final or fully accurate, when it might only capture one facet of a more complex reality.
- Problem: How do we prevent overconfidence in conceptual representations and keep them open to refinement as new insights are gained?
Representational flexibility vs. efficiency
- Challenge: Balancing the need for flexible, adaptive representations with the need for efficient processing. Overly flexible systems may become computationally heavy and slow.
- Problem: How do we design representations that are adaptable yet efficient, capable of handling complexity without overwhelming the system?
Conceptual drift over time
- Challenge: Concepts can drift as they evolve with new inputs or experiences. Keeping them coherent while allowing them to change without becoming detached from their original meaning is difficult.
- Problem: How do we manage conceptual drift in a way that ensures coherence over time while still allowing for natural evolution?
Integration of diverse representations
- Challenge: Different concepts and their representations may emerge from different domains (e.g., scientific, social, emotional). Integrating these into a unified model can be difficult without losing the richness of each individual domain.
- Problem: How do we create a system where diverse conceptual representations can coexist and interact meaningfully without oversimplifying?
Hierarchical vs. networked representations
- Challenge: Deciding between a hierarchical model (where concepts are organized by levels of importance or abstraction) versus a networked model (where concepts are connected in a web). Each approach has trade-offs.
- Problem: How do we combine hierarchical structure with networked flexibility, ensuring both clarity and interconnectivity?
Grounding abstract concepts
- Challenge: Abstract concepts are harder to represent because they often lack clear real-world counterparts or immediate experiential grounding.
- Problem: How do we ground abstract concepts in a meaningful way, ensuring they stay connected to reality while retaining their abstract value?
Temporal dynamics
- Challenge: Concepts evolve not only in structure but also over time as they interact with new information and experiences. Representations must account for this temporal fluidity.
- Problem: How do we represent the evolution of concepts over time, keeping track of temporal changes without losing their original meaning?