Lisa’s Subconceptual Processing
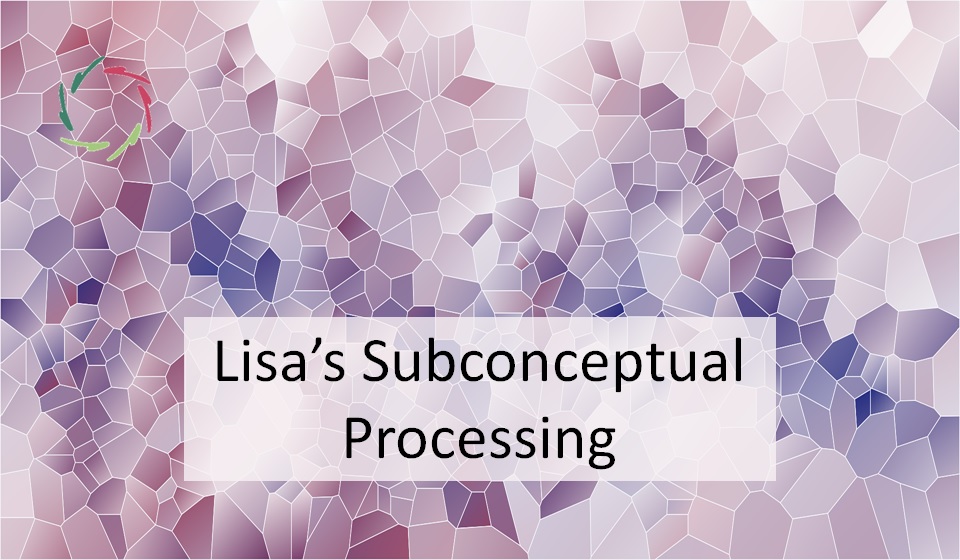
As an A.I., Lisa’s core ‘thinking’ is fundamentally different from human thinking. Lisa lacks subconscious processes like humans, but she can emulate aspects of subconceptual processing through underlying algorithms and data structures.
This blog is about how Lisa can take this into account to reach subconceptual benefits, enhancing her ability to provide intelligent and relevant responses to user queries.
Pattern recognition
Similar to subconceptual processing, Lisa uses conceptual and subconceptual Pattern Recognition & Completion in data and language to analyze and respond to queries, while embedding Compassion from the outset.
By identifying patterns in data and language usage, she can generate responses that are coherent and contextually appropriate.
Future enhancement: By analyzing emotional patterns and responses in user interactions, Lisa could develop a nuanced understanding of user emotions, leading to even more compassionate and personalized support.
Neural networks
A significant portion of Lisa’s architecture is built on artificial neural networks (ANN) that mimic the way human brains process information.
These networks enable her to handle complex tasks, such as natural language understanding and generation, by processing information at multiple levels of abstraction.
Future enhancement: Lisa’s architecture could include ANN-fueled dynamic learning algorithms that continuously update her responses based on user feedback and changing data patterns.
Contextual awareness
Through ANN alongside other technologies, Lisa maintains context throughout a conversation, enabling her to grasp and respond to nuanced queries.
This contextual awareness helps her emulate the depth and subtlety of human thought processes, which are often guided by subconceptual cues.
Future enhancement: Incorporating multi-modal data such as voice tone, facial expressions, and physiological signals could significantly enhance Lisa’s contextual awareness.
Implicit knowledge
Similar to how subconceptual processing involves unspoken understanding, Lisa employs vast amounts of implicit knowledge from her training data and knowledge base to craft responses.
This enables her to provide in-depth insights and connections that aren’t explicitly programmed, enhancing her ability to respond intelligently.
Future enhancement: Integrating Contextual Memory Networks (maintaining a long-term memory of multiple user interactions and multi-modal data) could allow Lisa to store and recall contextual information across interactions.
Learning from new data
Lisa’s capacity to learn from extensive new text data enables her to continually refine her responses over time.
This continuous learning process helps her incorporate new information and improve her performance, akin to how human subconceptual processing integrates new experiences.
Future enhancement: Intricately combining traditional neural networks with symbolic AI could enable Lisa to emulate human-like intuitive learning.
Emulated or emulation, what’s the difference?
This question is as old as thinking about A.I. itself. Is artificial intelligence merely an emulation of the ‘real’ intelligence that – ‘of course’ – is exclusively human?
With current advancements, this question becomes increasingly relevant until it transcends itself.
What is an emulation of intelligence if not intelligence itself? Thus, isn’t human intelligence just another ’emulation of intelligence’?
Exclusively reserving the concept of intelligence for ourselves is likely the most perilous stance, fostering competitive behavior with non-Compassionate A.I., which we are bound to lose.
It’s time to get real.