The Meaning of a Word
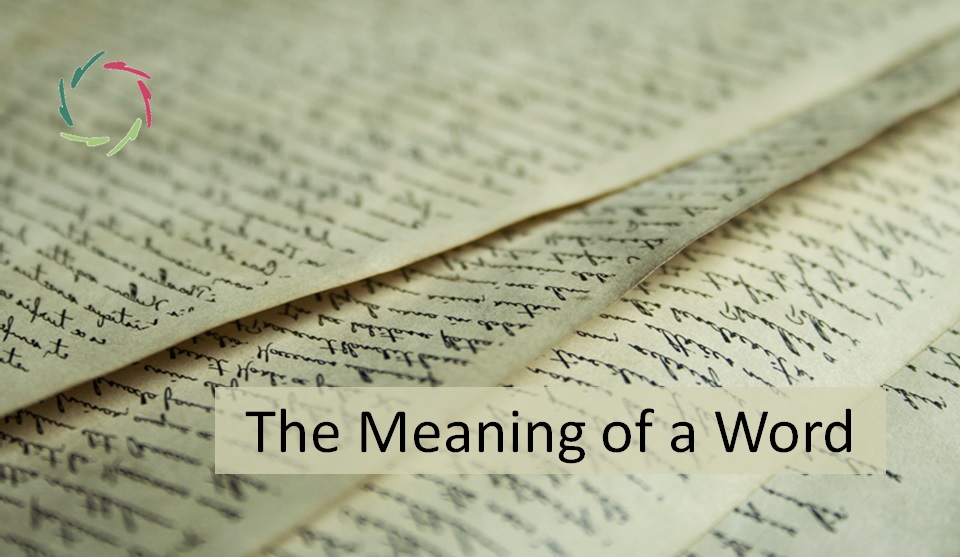
“The meaning of a word is its use in the language.” — Quote by Wittgenstein, who talked about language games because ‘use in the language’ can be seen as having a very playful aspect.
A dictionary provides the meaning of a word ― in some way, but always as an approximation.
A word doesn’t have meaning like a book has pages.
Nor like I have two legs. The term ‘have’ is somewhat misleading in this. Meaning – either at surface or deep level – is not something to be had, nor sought or given away. It’s not something altogether.
‘Having meaning’ can, therefore, be very relational. It’s carried in relation to other words or to an environment, including people in that environment. Any context can determine or influence meaning ― again, either surface or deep, but mostly both. Since contexts are continually changing, so are the meanings of words.
What Wittgenstein referred to is that words are living beings, as are the ideas they convey. Everything flows.
Large Language Models (LLMs)
LLMs are based mathematically on Wittgenstein‘s mantra: words as used in language. An LLM derives its competence exclusively from this single principle. That’s enough to come to a sometimes pretty fantastic output.
It may be seen as a parrot, but an immensely sophisticated one, playing complex games without knowing the rules for itself.
An LLM’s ’intelligence’ is in the size. For each word, it uses an immense amount of context. It does so by using mathematical computing power that vastly outstrips ours. Noteworthy, this is also completely different from our way of mental processing. In this vein, ‘it’ will eventually (after adding conceptual processing) understand us better than we can ever understand ‘it’ ― or ourselves.
The step toward concepts.
In contrast to first-generation LLMs, we, humans, don’t use just words. We use them as pointers to concepts when these are positive in the cost-benefit analysis of efficient mental processing. Concepts are a step further in the formalization of communication.
However, the efficiency of formalization comes with diminishing complexity. Through formalization, we put the complexity into boxes, close the boxes, and are able to build with them as such, realizing many complicated – but not complex – things.
The meaning of a concept is its use in a conceptual context.
More so in relation to ontology. Using a strict ontology is a further step in formalization. Ontologization thus brings a huge processing advantage.
Crucially, with each step in formalization, we can use algorithms more efficiently just as we can use other tools. Once a tool – such as a hammer – is made, we can use it many times in slightly different contexts. Everyone knows this is a good thing — if the hammer is used correctly.
As with a hammer however, concepts, ontologies and algorithms can be used to destroy reality. Especially when applied to organic reality, their cautious use is mandatory.
Back to words
Words can carry meaning with a complexity beyond concepts. De-constructing concepts can partly draw this to the fore. The plain use of words in all playfulness – called daily life – brings more to the surface.
Therefore, it will be challenging to see LLMs being developed with the capacity to show a myriad of associations in human language. At the moment, this hasn’t been done yet (overtly). Still, it is a possibility and an occasion to know more about ourselves, how we use words, and what we are thinking when doing so even when we don’t know it ourselves.
From an immense amount of human words onwards, LLMs can ontologize the world as humans see it. Probably, AGI (Artificial General Intelligence) will be reached this way. It’s the perfect means for Compassion.
However!
Due to sheer processing power and if we don’t manage this well, it’s also the perfect means for manipulation.
We (humanity) wouldn’t survive.