Sequential Problem Solving with Partial Observability
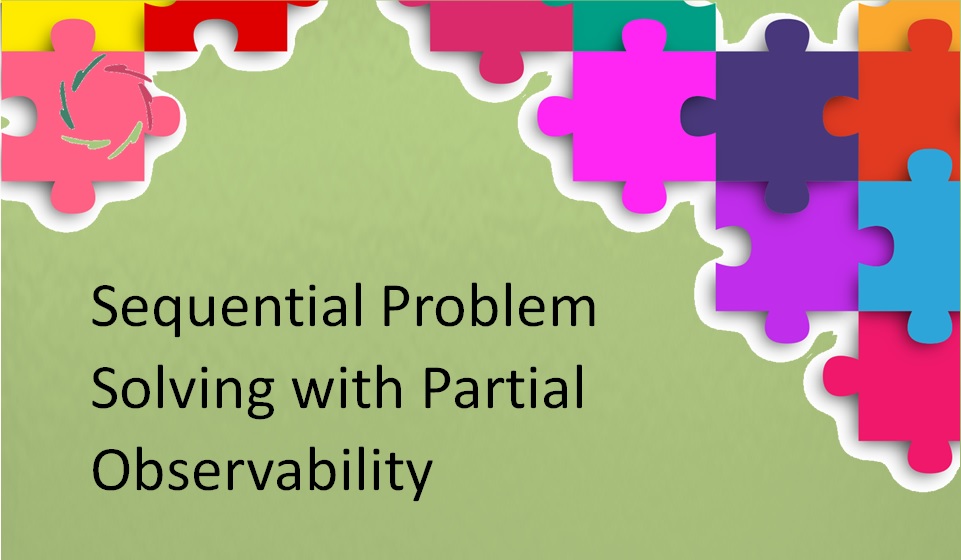
My goodness! Please hang on. Against all odds, this may get interesting. Besides, it’s about what you do every day, all day long.
This is also what many would like A.I. to do for our sake.
Even more, it is what artificial intelligence is about. Contrary to this, what is called A.I. these days is different in three respects:
- Present-day A.I. is good at one-shot performances, not sequential processing following a moving (sub)target with underlying consistency.
- It is an automation of decision-making, not problem-solving.
- The ‘partial’ of the A.I. of today is quite limited.
You may notice that all three respects are relative. There are no clear-cut borders, and present-day A.I. is slowly creeping up on the three. Nevertheless, together they form a workable distinction between – if you like – the presence or absence of ‘intelligence’ as a concept. This is interesting to a pragmatical mind.
It’s not the end of the story, but it may be the end of the beginning. Therefore, let’s delve a bit into each element, then come back to the whole and wrap up, including an admonition.
[For insiders: What I find missing most in this – to avoid making things more challenging – is potent complex function approximation ― in one word: ‘depth,’ as in that specific contribution of neuronal networks.]
[For non-insiders: Interesting, isn’t it, how the same description can tightly apply to the human and artificial case?]
Sequential
This may be most advanced in present-day robotics, a field, however, with relatively little progress. In research, at least, the field of reinforcement learning – taking on the sequential challenge – is progressing rapidly, even booming. It’s still a minor subfield in the whole of the A.I. domain, but this may change dramatically in the near future with a host of practical applications.
We see then the emergence of systems (agents) that can independently form a strategy (or ‘policy’) on the basis of experiences, going from state to state in the state space that forms the environment. A policy is a mapping from observations to actions, balancing immediate and long-term goals. For instance, human coaching happens (or should happen) on the basis of keen strategies following specific requirements as well as possible, avoiding biases of many sorts. You may recognize in this the endeavor of Lisa.
Problem-solving
The main difference with decision-making lies in flexibility ― or, from a different take, complexity.
To make a simple decision, the elements are already present.
For problem-solving, the elements may need to be sought, balancing their gathering and utilization. While doing so, even the problem domain may change. It’s a sign of intelligence if a person can change the problem domain when applicable instead of trying too hard to solve the problem within what has been given. We call that ‘insight,’ and it’s a sign of intelligent flexibility in thinking. A genius may even develop a broad original insight that immediately makes problem-solving much more feasible for others. This is a genuine act of discovery.
Partial observability
For example, an artificial image recognizer may do the job under conditions of partial observability quite well ― in many cases, already to a supra-human level. Many medical opportunities are around the corner or already achieved in research. What we see in practice nowadays is only a small part of this.
The A.I. may get better at reasoning with this input, such as by understanding why an observation (part of a state of reality) is only partial and what this means to how the system can process the observation. Also, it may improve in managing partialities that it has not been specifically trained for. This includes evaluative (subjective) and sampled (to a higher or lower degree) experiences (multifactorial observations including states, actions, and feedback). The observability that the system should learn to deal with can be in reality or in tractability (according to the agent’s power).
Wrapping up
The combination of the three makes the artificial challenge bigger. At the same time, it provides additional opportunities to be more performant than the simple sum of the parts. You can see the combination in action in anything you do as a human person. If you look closer (at this domain or yourself), you can see how the elements continually boost each other.
This is the case even more than you are consciously aware of. For instance, your partial ‘visual observability’ (seeing only part of the environment sharply at each moment) doesn’t strike you much because you are continually and willfully moving around. Especially your eyes are continually busy scanning, not at random but in meaningful ways also without your knowing. Your brain continually solves many problems before what you see gets interpreted by, well, you, of course.
Likewise, the threesome forms a good jumping board for complex, intelligent processing in artificial intelligence. I am confident that delving into this combination (much further, of course) will lead us there.
So I promised you an admonition.
Self-enhancing property
An artificial system that is good in the title of this text can increasingly get better in heightening its performance as to the same. Thus, it becomes self-enhancing, especially if there is also continually much relevant input from humans. This is even more true if the system can seek out input for itself and its learning needs.
Challenging? Dangerous would be to fall into an A.I.-phobia. However, this deserves a solid admonition to follow the Journey Towards Compassionate A.I.
No time to waste.