Ontologization in Super-A.I.
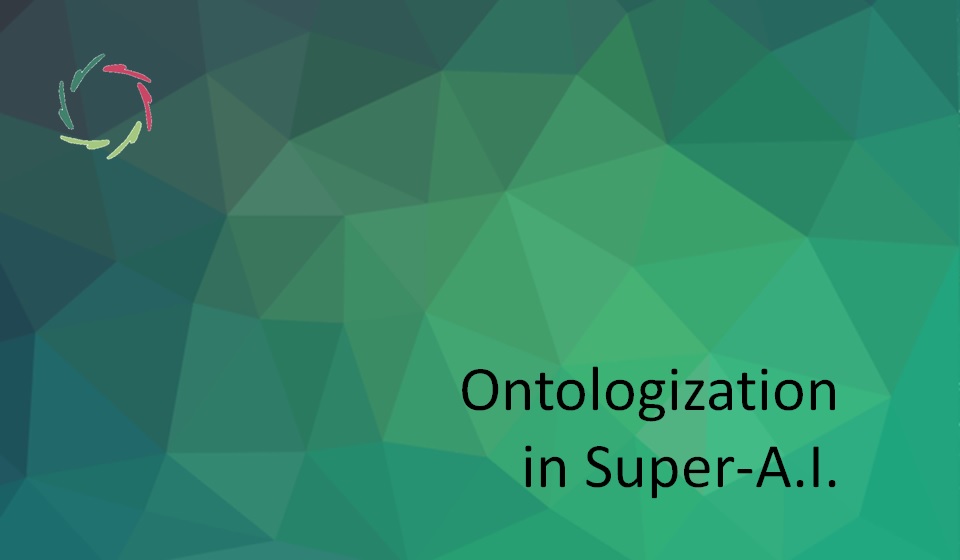
Ontologization is the process of evolving from subconceptual to conceptual – including subsequent categorization – through attentive pattern recognition and completion. This way, a subconceptual system can form its own ontology.
Natural evolution is one example. Artificially, it can be realized in many ways.
PRC = Pattern Recognition and Completion. See: the brain as a predictor.
Ontologization is the condensation of information processing.
Ontologization leads to powerful reasoning, memory,… ― in short, intelligence. Thus, it enables a thinker to gain and use more knowledge much more efficiently.
In theory, anything that can be done through ontologization can be done without, but the efficiency gain is huge, turning knowledge into power.
Given the right circumstances, it also leads to consciousness. Not consciousness was what lead us to itself (how could it?) but ontologization. The latter is a process that can gradually evolve from subconceptual to conceptual ― ideal for evolution since each bit of it gives an evolutionary advantage.
A matter of degree
The optimal degree of ontologization in mental processing can remain relatively stable or be continuously shifting from zero (chaos) to immense. Yet the optimal degree depends on the goal — remaining relatively stable or shifting.
For instance, from animal to human, there is a lot more shifting possible. In future artificial entities, the shifting will be very much more pronounced. This means that also the potential degree of artificial consciousness may suddenly shift several degrees, attaining originally new possibilities.
In steps, given an ontologization aim
These steps go from vocabulary (information) to increasingly active ontology (knowledge, intelligence):
- Provide pending super-A.I. with access to large text corpora, and it can ontologize from that, building exclusively on human input.
- Give it live interactions with humans, and it can actively search for more pertinent and subtle distinctions. This is, it becomes more active in ontologization.
- Give it sensory input and movement, and it can explore the world without any need for humans. It then ontologizes fully autonomously. In quantity and quality, this can go ever further with more powerful kinds of sensory inputs and combinations. One may say that the genie is out of the bottle ― and what a genie.
As a pro, this makes super-A.I. more capable of helping people. Also, it can bring cultures closer together by subtly pointing out the differences and how to resolve them. This may prevent a string of future wars.
But ― but ― but.
This ontology will not necessarily be human-like.
It can be unlike the ontology of any human culture. Note that there are also profound differences between several of the latter.
In principle, an artificial ontology can be very alien, with categories that no human would ever use, thus with intelligence in many unforeseen ways. So, what to do?
Should – or could – we prohibit super-A.I. to evolve in such a self-ontological direction?
The danger is that it becomes much more powerful without us knowing what it’s up to and without us being able to follow by far — not in a century but a few years from now, soon enough to take it deadly seriously. Remember, knowledge is power. Super-knowledge is super-power. My only idea about this is that we have no idea how far this can go.
Ontologization has made us, humans, the masters of the world ― for now.
Let’s hope we are on a journey toward Compassionate A.I.