Active Learning in A.I.
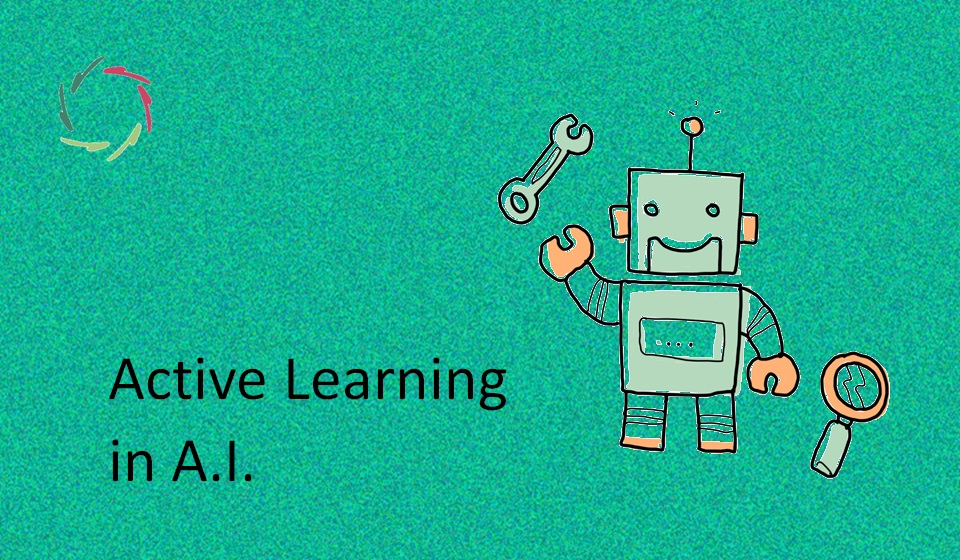
An active learner deliberately searches for information/knowledge to become smarter.
In biological evolution on Earth
The ‘Cambrian explosion’ was probably jolted by the appearance of active learning in natural evolution. It was the time when living beings started to chase other living beings— thus also being chased, heightening the challenges of survival.
This mutual predation pressure meant they needed to become more intelligent and quickly learn about ever-changing environments. To do so, they needed to find out its essential aspects, again and again.
From then on until now (us), the stage was set.
Steps toward active learning in A.I.
Depending on the concept of ‘learning,’ one may see the simplest things in the digital world as steps toward active learning. For instance, the user interface to a database may be seen as a focused invitation for the user to enter data.
From here, one can see many steps toward the real thing. For instance, in algorithmic machine learning, the data input may be used by the system to come up with better inferences.
Skipping things like supervised learning in neural networks, unsupervised learning – as implied by the name – requires less active mingling from outside to come up with interesting new patterns. One can say more or less that the system has indeed actively learned these patterns.
Another step lies in Reinforcement Learning, where the system can ‘actively’ learn on the basis of rewards (as do we).
‘Active learning’ is clearly not a matter of all or nothing.
The real thing
In this respect, ‘active’ means that the system knows what to do to learn what it wants to learn and that it can take the initiative to go for it. One can see this in humans – and other animals – from childhood. Remember the Cambrian era.
In A.I., talking about ‘knowing’ and ‘wanting’ is less evident, of course. In this sense, we can talk about it to the degree that it looks like it. We can think about ‘A.I.-knowing’ and ‘A.I.-wanting.’ This enables us to talk about ‘active learning in A.I.’ as a valid concept ― one kind of active learning. The human way is another kind with some comparable features.
Some features/enablers of active learning
Probably the most prominent of these is ontologization and conceptual thinking. Concepts are tools that make thinking much more efficient by leaving out many irrelevant details. This enables the thinker to bootstrap his thinking to higher levels. He can think more conceptually about his thinking, his learning, what he needs to learn, and how to go for it, learning exploratively. Each of these elements is another feature of active learning — with continua all the way through.
Conceptual thinking also enables the use of analogy, thus an even higher level of active learning from one domain to another — including learning how to learn better.
Is active learning a breakthrough toward real A.I.?
In view of the above, I would answer positively. If A.I. attains the real thing of active learning, it becomes the real thing of intelligence IF we don’t want to reserve these terms for ourselves (which would be dangerously misleading).
An actively learning A.I. can ask us, or the Internet, or the world itself, for anything it wants to know and principally without needing our initiative. This sets in motion a singular whirlpool of ever more learning and knowing.
Making us redundant.
This is why we need the Journey Towards Compassionate A.I.
Since a year ago, I see no other decent solution.