From Compression to Prediction
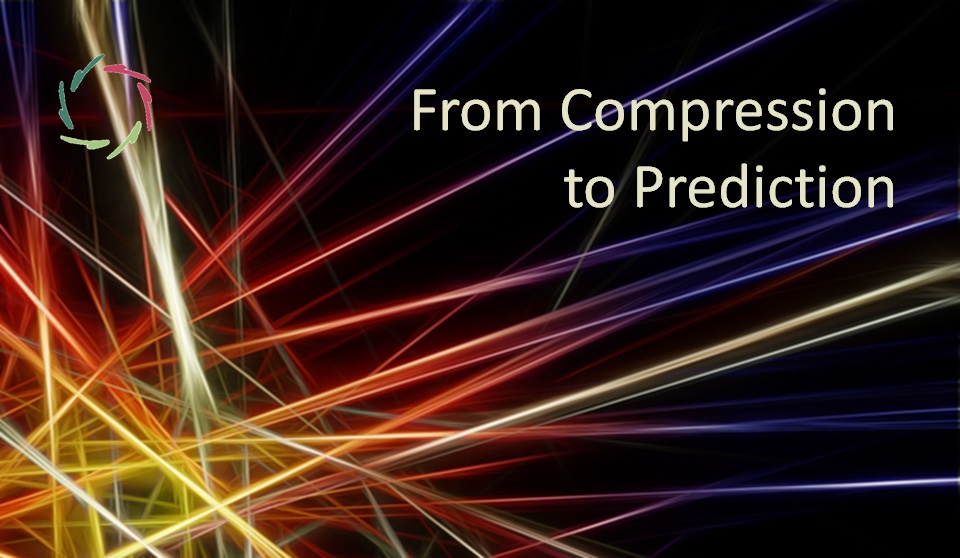
Compressing information can intriguingly lead to enhanced predictive capabilities. This general scheme is recognizable in many contexts ― organic and artificial.
Life itself
Life can be understood as a local defense against universal entropy (chaos or heightening of chaos). Within any bubble of life, there is a concentration of resources to this aim. At the same time, any form of life needs to be predictive, ‘knowing’ how to respond to specific circumstances in order not to lose the battle against entropy.
From bacterium to Bach, in order to stay alive and thrive, the organism must therefore ‘know’ how to predict and respond in ever-changing circumstances.
Intelligence
The brain/mind is one giant pattern recognizer. Internally, mappings go on from patterns to patterns and from brain parts to brain parts. At each level, information is compressed. This functions as a brainy prediction-then-correction of what is supposed to be the output from one level to another.
The scheme is repeated at the level of the whole brain and of the organism. A massive amount of input (much more than we consciously perceive) is compressed into a tiny information space from which more or less appropriate actions result.
Thus, organic intelligence follows the general scheme throughout evolution.
Large language models (LLMs)
Perhaps unsurprisingly, the general scheme continues into the artificial world.
LLMs are basically a vectorization (parameterization, numberification) of massive amounts of human-produced text. The text is compressed into the numbers while much (but not all) of the information is retained, albeit for the human eye and mind in an implicit manner.
The LLM advantage is that a computer can – through the huge power of number crunching – more easily present the information in new ways that are understandable to humans. Notoriously, this has recently been the case in suprisingly many fields and to a surprising degree even to the developers themselves.
As such, there is no humanly explicit knowledge within the numbers. Not any single number stands for a concept. Nevertheless, the computer can predict the next word in a humanly sentence fairly accurately — mostly. All output of an LLM is a prediction, as is all output of the human brain.
In both cases, the predictions seem pretty intelligent, although necessarily only to a certain degree. In neither of both cases do we exactly know how it’s done.
Relevantly lossy information compression by itself seems to lead to the spontaneous emergence of predictive capability.
As in any setting, there is no emergence of information that is not already inherently – implicitly – present. The mechanism of compression can then be relatively simple, of course, because the resulting intelligence doesn’t come from this mechanism but from the information.
It’s as if the compression puts some pressure on the system through which, time and again, some Gestalt comes forward. These Gestalts must have entered the system through its contact with reality.
Hm, is there eventually no need for a deeper level of comprehension?
Does this mean that combinations of LLMs together with other technologies will surpass our human intelligence pretty soon?
These are pretty crucial questions.